For the Love of Chatbots
Over the past few months I have been collecting the best resources on NLP and how to apply NLP and Deep Learning to Chatbots.
Every
once in awhile, I would run across an exception piece of content and I
quickly started putting together a master list. Soon I found myself
sharing this list and some of the most useful articles with developers
and other people in bot community.
In
process, my list became a Guide and after some urging, I have decided
to share it or at least a condensed version of it -for length reasons.
This
guide is mostly based on the work done by Denny Britz who has done a
phenomenal job exploring the depths of Deep Learning for Bots. Code
Snippets and Github included!
Without further ado… Let us Begin!
DEEP LEARNING FOR CHATBOTS OVERVIEW
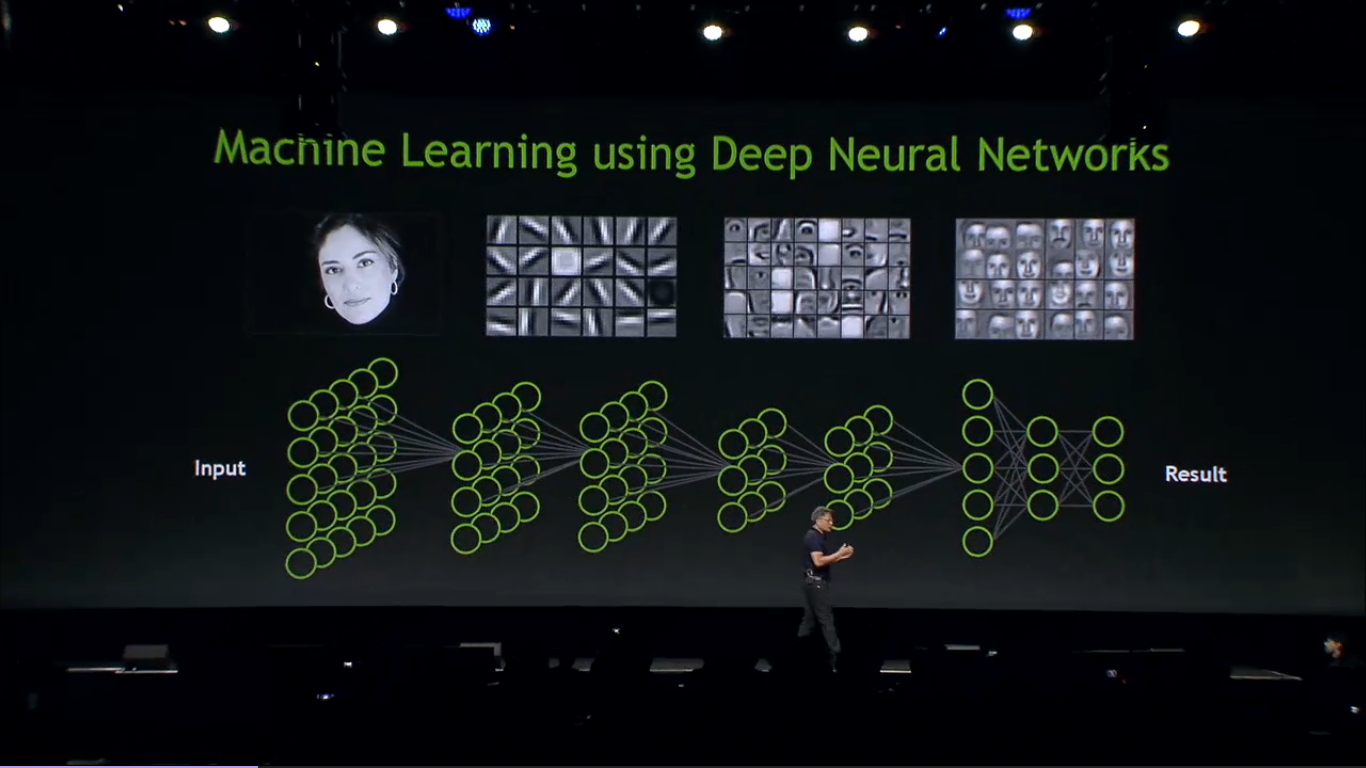
Chatbots,
are a hot topic and many companies are hoping to develop bots to have
natural conversations indistinguishable from human ones, and many are
claiming to be using NLP and Deep Learning techniques to make this
possible. But with all the hype around AI it’s sometimes difficult to
tell fact from fiction.
In
this series I want to go over some of the Deep Learning techniques that
are used to build conversational agents, starting off by explaining
where we are right now, what’s possible, and what will stay nearly
impossible for at least a little while.
If you find this article interesting you can let me know here.
A TAXONOMY OF MODELS
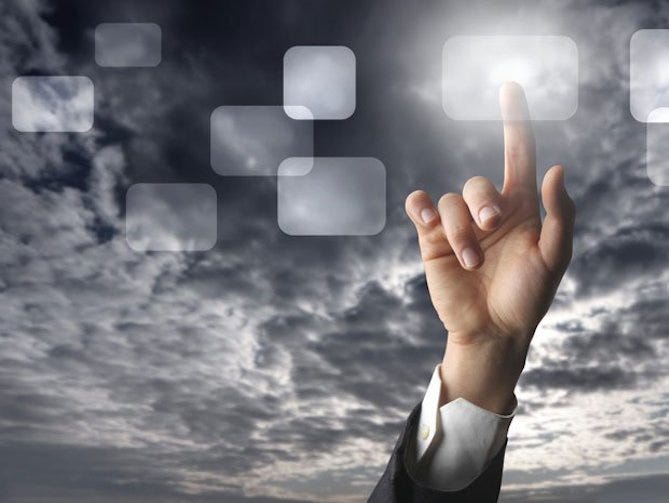
RETRIEVAL-BASED VS. GENERATIVE MODELS
Retrieval-based models (easier)
use a repository of predefined responses and some kind of heuristic to
pick an appropriate response based on the input and context. The
heuristic could be as simple as a rule-based expression match, or as
complex as an ensemble of Machine Learning classifiers. These systems
don’t generate any new text, they just pick a response from a fixed set.
Generative models (harder)
don’t rely on pre-defined responses. They generate new responses from
scratch. Generative models are typically based on Machine Translation
techniques, but instead of translating from one language to another, we
“translate” from an input to an output (response).
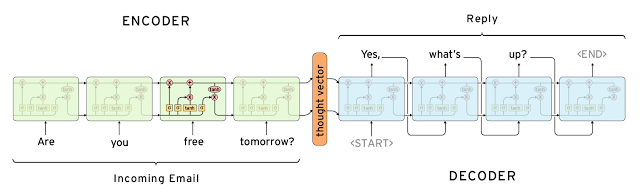
Both
approaches have some obvious pros and cons. Due to the repository of
handcrafted responses, retrieval-based methods don’t make grammatical
mistakes. However, they may be unable to handle unseen cases for which
no appropriate predefined response exists. For the same reasons, these
models can’t refer back to contextual entity information like names
mentioned earlier in the conversation. Generative models are “smarter”.
They can refer back to entities in the input and give the impression
that you’re talking to a human. However, these models are hard to train,
are quite likely to make grammatical mistakes (especially on longer
sentences), and typically require huge amounts of training data.
Deep
Learning techniques can be used for both retrieval-based or generative
models, but research seems to be moving into the generative direction.
Deep Learning architectures likeSequence to Sequence
are uniquely suited for generating text and researchers are hoping to
make rapid progress in this area. However, we’re still at the early
stages of building generative models that work reasonably well. Production systems are more likely to be retrieval-based for now.
LONG VS. SHORT CONVERSATIONS
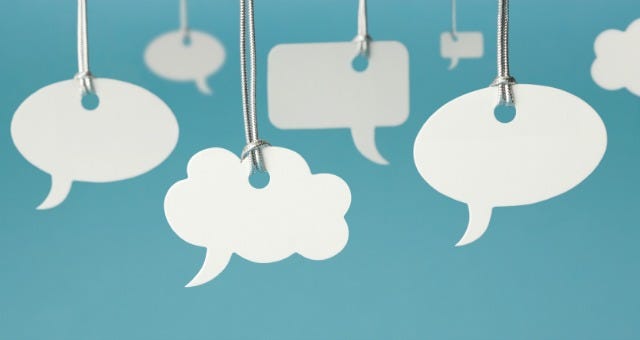
The longer the conversation the more difficult to automate it. On one side of the spectrum areShort-Text Conversations (easier)
where the goal is to create a single response to a single input. For
example, you may receive a specific question from a user and reply with
an appropriate answer. Then there are long conversations (harder)
where you go through multiple turns and need to keep track of what has
been said. Customer support conversations are typically long
conversational threads with multiple questions.
OPEN DOMAIN VS. CLOSED DOMAIN
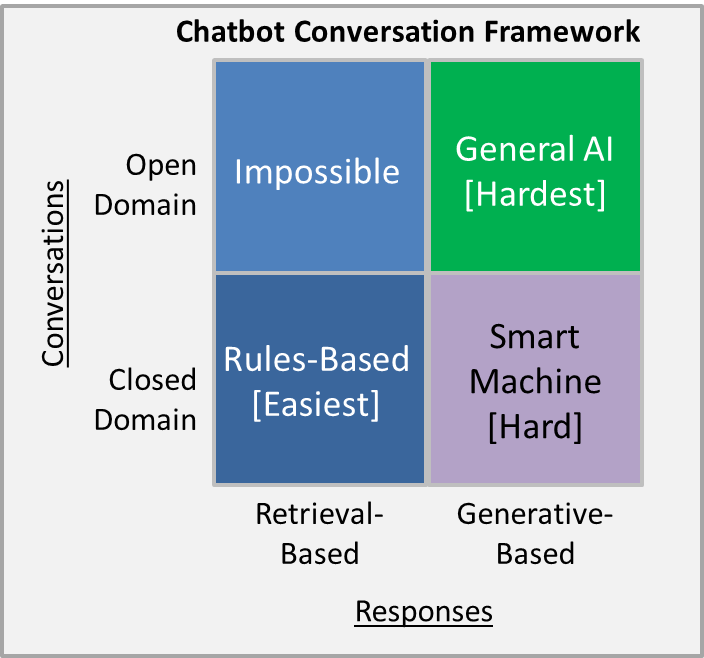
In an open domain (harder)
setting the user can take the conversation anywhere. There isn’t
necessarily have a well-defined goal or intention. Conversations on
social media sites like Twitter and Reddit are typically open
domain — they can go into all kinds of directions. The infinite number
of topics and the fact that a certain amount of world knowledge is
required to create reasonable responses makes this a hard problem.
“Open Domain: I can ask a question about any topic… and expect a relevant response. (Harder) Think of a long conversation around refinancing my mortgage where I could ask anything.” Mark Clark
In a closed domain (easier)
setting the space of possible inputs and outputs is somewhat limited
because the system is trying to achieve a very specific goal. Technical
Customer Support or Shopping Assistants are examples of closed domain
problems. These systems don’t need to be able to talk about politics,
they just need to fulfill their specific task as efficiently as
possible. Sure, users can still take the conversation anywhere they
want, but the system isn’t required to handle all these cases — and the
users don’t expect it to.
“Closed Domain: You can ask a limited set of questions on specific topics. (Easier). What is the Weather in Miami?”
“Square 1 is a great first step for a chatbot because it is contained, may not require the complexity of smart machines and can deliver both business and user value.
Square 2, questions are asked and the Chatbot has smart machine technology that generates responses. Generated responses allow the Chatbot to handle both the common questions and some unforeseen cases for which there are no predefined responses. The smart machine can handle longer conversations and appear to be more human-like. But generative response increases complexity, often by a lot.
The way we get around this problem in the contact center today is when there is an unforeseen case for which there is no predefined responses in self-service, we pass the call to an agent.” Mark Clark
COMMON CHALLENGES
There
are some obvious and not-so-obvious challenges when building
conversational agents most of which are active research areas.
INCORPORATING CONTEXT
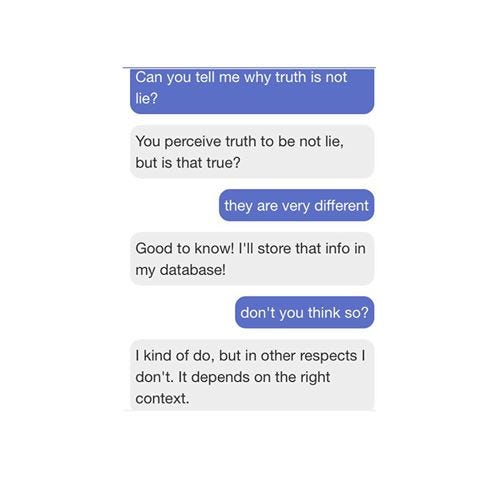
To produce sensible responses systems may need to incorporate both linguistic context andphysical context.
In long dialogs people keep track of what has been said and what
information has been exchanged. That’s an example of linguistic context.
The most common approach is toembed the conversation into a vector, but doing that with long conversations is challenging. Experiments in Building End-To-End Dialogue Systems Using Generative Hierarchical Neural Network Models and Attention with Intention for a Neural Network Conversation Model
both go into that direction. One may also need to incorporate other
kinds of contextual data such as date/time, location, or information
about a user.
COHERENT PERSONALITY
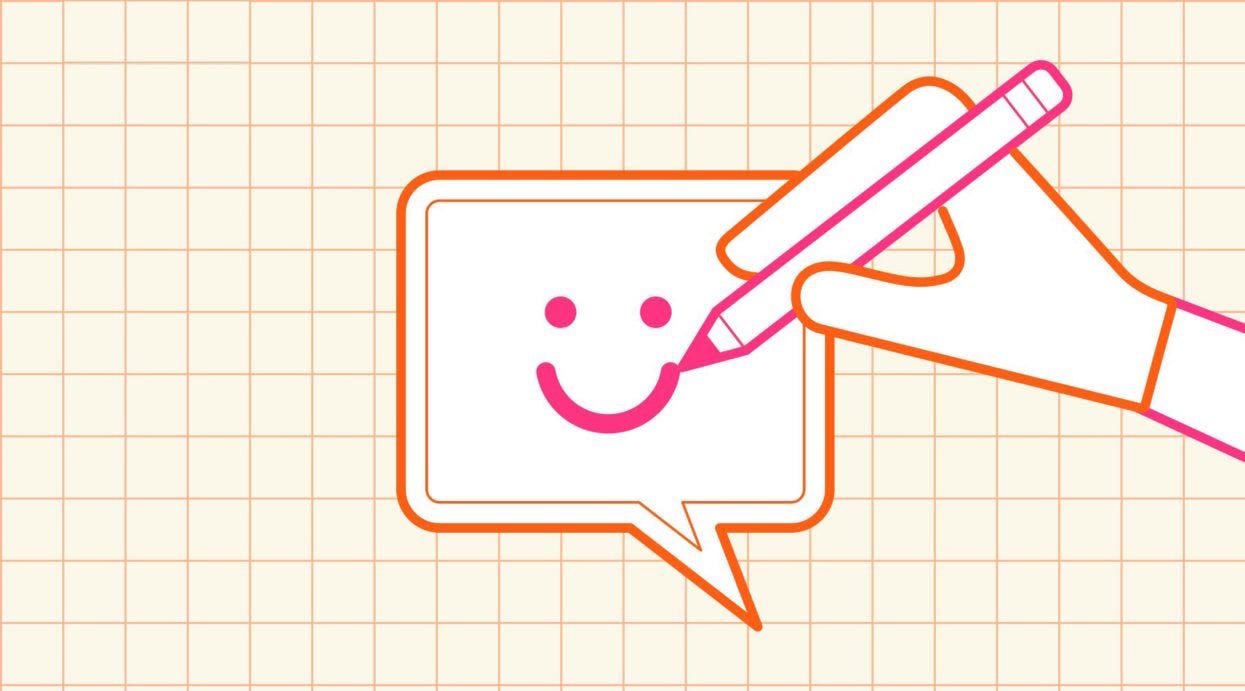
When
generating responses the agent should ideally produce consistent
answers to semantically identical inputs. For example, you want to get
the same reply to “How old are you?” and “What is your age?”. This may
sound simple, but incorporating such fixed knowledge or “personality”
into models is very much a research problem. Many systems learn to
generate linguistic plausible responses, but they are not trained to
generate semantically consistent ones. Usually that’s because they are
trained on a lot of data from multiple different users. Models like that
in A Persona-Based Neural Conversation Model are making first steps into the direction of explicitly modeling a personality.
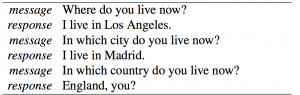
EVALUATION OF MODELS
The
ideal way to evaluate a conversational agent is to measure whether or
not it is fulfilling its task, e.g. solve a customer support problem, in
a given conversation. But such labels are expensive to obtain because
they require human judgment and evaluation. Sometimes there is no
well-defined goal, as is the case with open-domain models. Common
metrics such as BLEUthat
are used for Machine Translation and are based on text matching aren’t
well suited because sensible responses can contain completely different
words or phrases. In fact, in How NOT To Evaluate Your Dialogue System: An Empirical Study of Unsupervised Evaluation Metrics for Dialogue Response Generation researchers find that none of the commonly used metrics really correlate with human judgment.
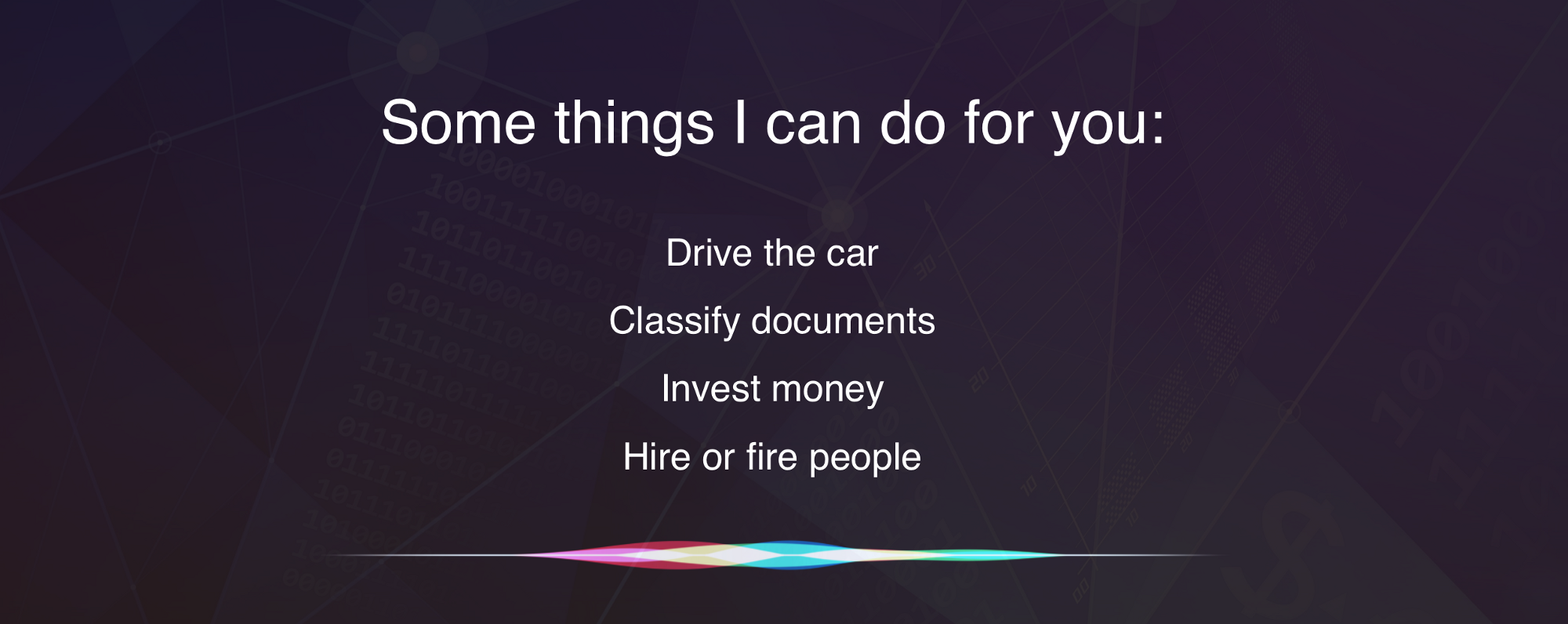
INTENTION AND DIVERSITY
A
common problem with generative systems is that they tend to produce
generic responses like “That’s great!” or “I don’t know” that work for a
lot of input cases. Early versions of Google’s Smart Reply tended to respond with “I love you”
to almost anything. That’s partly a result of how these systems are
trained, both in terms of data and in terms of actual training
objective/algorithm. Some researchers have tried to artificially promote diversity through various objective functions.
However, humans typically produce responses that are specific to the
input and carry an intention. Because generative systems (and
particularly open-domain systems) aren’t trained to have specific
intentions they lack this kind of diversity.
HOW WELL DOES IT ACTUALLY WORK?
Given
all the cutting edge research right now, where are we and how well do
these systems actually work? Let’s consider our taxonomy again. A
retrieval-based open domain system is obviously impossible because you
can never handcraft enough responses to cover all cases. A generative
open-domain system is almost Artificial General Intelligence (AGI)
because it needs to handle all possible scenarios. We’re very far away
from that as well (but a lot of research is going on in that area).
This
leaves us with problems in restricted domains where both generative and
retrieval based methods are appropriate. The longer the conversations
and the more important the context, the more difficult the problem
becomes.
In a recent interview, Andrew Ng, now chief scientist of Baidu, puts it well:
Most of the value of deep learning today is in narrow domains where you can get a lot of data. Here’s one example of something it cannot do: have a meaningful conversation. There are demos, and if you cherry-pick the conversation, it looks like it’s having a meaningful conversation, but if you actually try it yourself, it quickly goes off the rails.
Many
companies start off by outsourcing their conversations to human workers
and promise that they can “automate” it once they’ve collected enough
data. That’s likely to happen only if they are operating in a pretty
narrow domain — like a chat interface to call an Uber for example.
Anything that’s a bit more open domain (like sales emails) is beyond
what we can currently do. However, we can also use these systems to
assist human workers by proposing and correcting responses. That’s much
more feasible.
Grammatical
mistakes in production systems are very costly and may drive away
users. That’s why most systems are probably best off using
retrieval-based methods that are free of grammatical errors and
offensive responses. If companies can somehow get their hands on huge
amounts of data then generative models become feasible — but they must
be assisted by other techniques to prevent them from going off the rails
like Microsoft’s Tay did.
IMPLEMENTING A RETRIEVAL-BASED MODEL IN TENSORFLOW
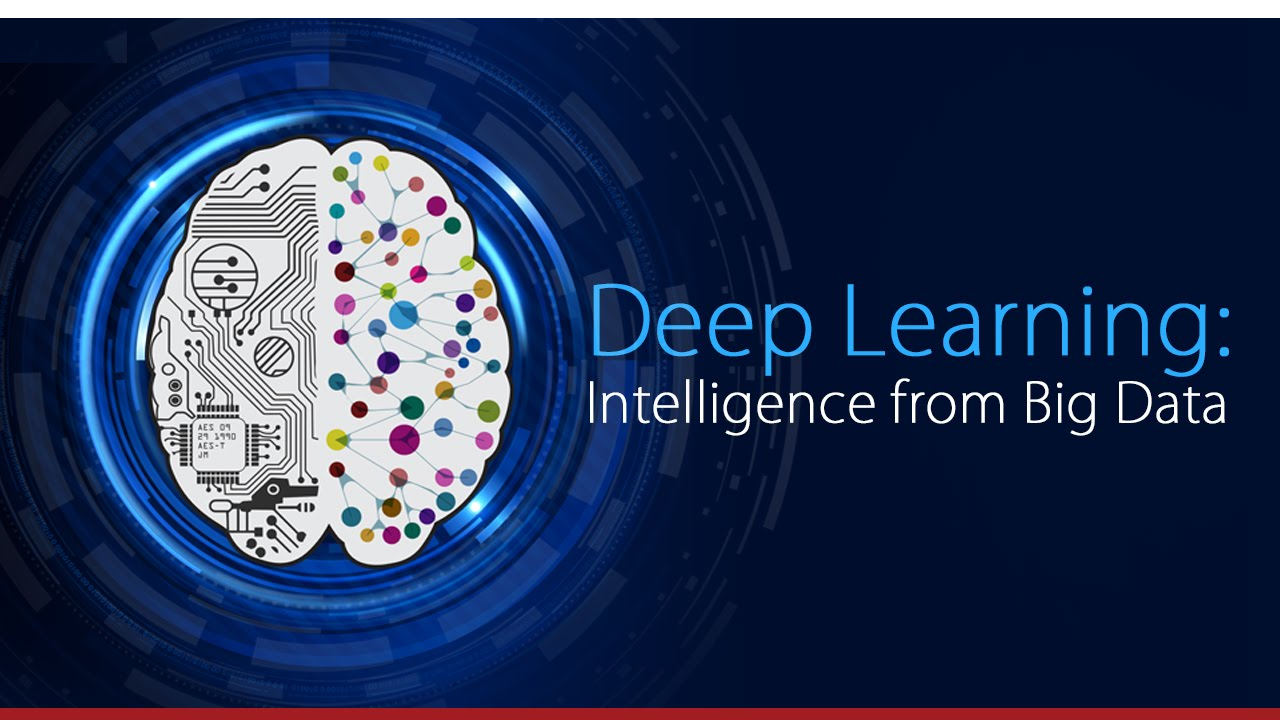
RETRIEVAL-BASED BOTS
The vast majority of production systems today are retrieval-based, or a combination of retrieval-based and generative. Google’s Smart Reply
is a good example. Generative models are an active area of research,
but we’re not quite there yet. If you want to build a conversational
agent today your best bet is most likely a retrieval-based model.
If you want me to write more articles like this, please let me know here.
THE UBUNTU DIALOG CORPUS
In this post we’ll work with the Ubuntu Dialog Corpus (paper, github).
The Ubuntu Dialog Corpus (UDC) is one of the largest public dialog
datasets available. It’s based on chat logs from the Ubuntu channels on a
public IRC network. The paper
goes into detail on how exactly the corpus was created, so I won’t
repeat that here. However, it’s important to understand what kind of
data we’re working with, so let’s do some exploration first.
The training data consists of 1,000,000 examples, 50% positive (label 1) and 50% negative (label 0). Each example consists of a context, the conversation up to this point, and an utterance,
a response to the context. A positive label means that an utterance was
an actual response to a context, and a negative label means that the
utterance wasn’t — it was picked randomly from somewhere in the corpus.
Here is some sample data.
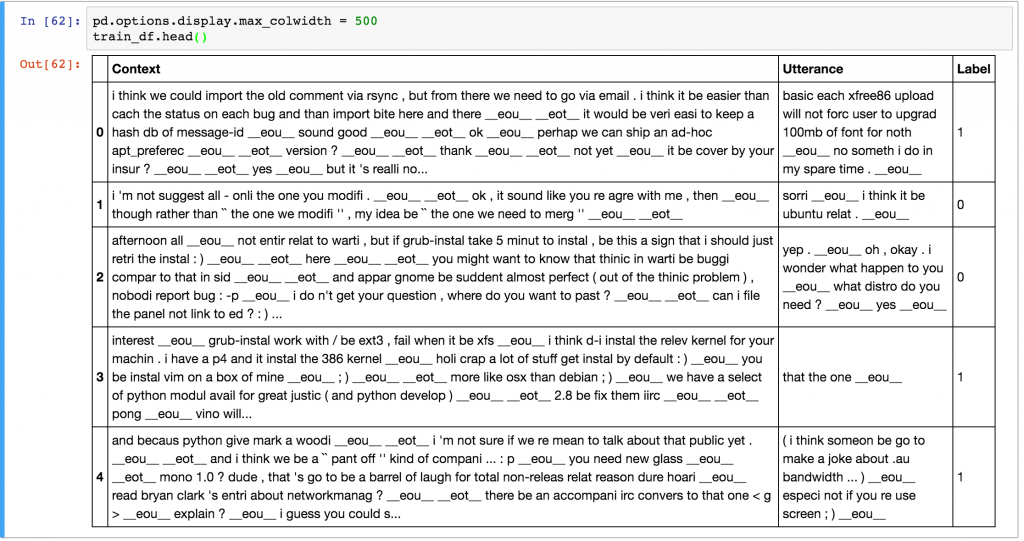
Note that the dataset generation script has already done a bunch of preprocessing for us — it hastokenized, stemmed, and lemmatized the output using the NLTK tool.
The script also replaced entities like names, locations, organizations,
URLs, and system paths with special tokens. This preprocessing isn’t
strictly necessary, but it’s likely to improve performance by a few
percent. The average context is 86 words long and the average utterance
is 17 words long. Check out the Jupyter notebook to see the data analysis.
The
data set comes with test and validations sets. The format of these is
different from that of the training data. Each record in the
test/validation set consists of a context, a ground truth utterance (the
real response) and 9 incorrect utterances called distractors. The goal of the model is to assign the highest score to the true utterance, and lower scores to wrong utterances.
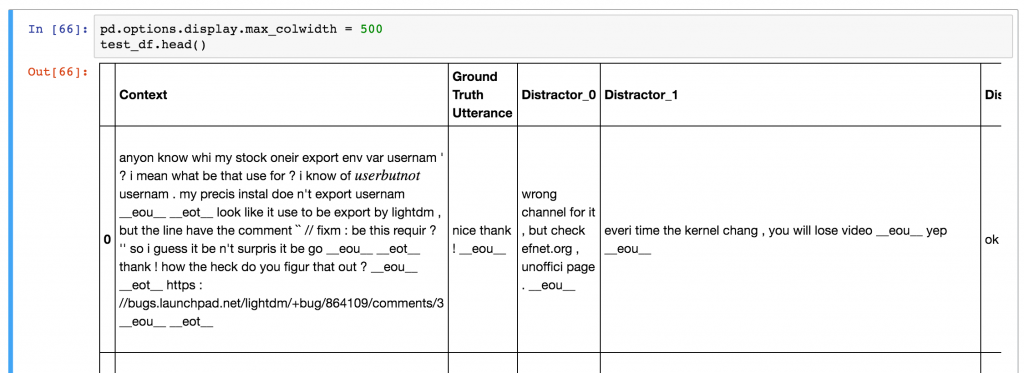
The are various ways to evaluate how well our model does. A commonly used metric is recall@k.
Recall@k means that we let the model pick the k best responses out of
the 10 possible responses (1 true and 9 distractors). If the correct one
is among the picked ones we mark that test example as correct. So, a
larger k means that the task becomes easier. If we set k=10 we get a
recall of 100% because we only have 10 responses to pick from. If we set
k=1 the model has only one chance to pick the right response.
At
this point you may be wondering how the 9 distractors were chosen. In
this data set the 9 distractors were picked at random. However, in the
real world you may have millions of possible responses and you don’t
know which one is correct. You can’t possibly evaluate a million
potential responses to pick the one with the highest score — that’d be
too expensive. Google’sSmart Reply uses clustering techniques to come up with a set of possible responses to choose from first. Or, if you only have a few hundred potential responses in total you could just evaluate all of them.
BASELINES
Before
starting with fancy Neural Network models let’s build some simple
baseline models to help us understand what kind of performance we can
expect. We’ll use the following function to evaluate our recall@k
metric:
def evaluate_recall(y, y_test, k=1):
num_examples = float(len(y))
num_correct = 0
for predictions, label in zip(y, y_test):
if label in predictions[:k]:
num_correct += 1
return num_correct/num_examples
Here,
y is a list of our predictions sorted by score in descending order, and
y_test is the actual label. For example, a y of [0,3,1,2,5,6,4,7,8,9]
Would mean that the utterance number 0 got the highest score, and
utterance 9 got the lowest score. Remember that we have 10 utterances
for each test example, and the first one (index 0) is always the correct
one because the utterance column comes before the distractor columns in
our data.
Intuitively,
a completely random predictor should get a score of 10% for recall@1, a
score of 20% for recall@2, and so on. Let’s see if that’s the case.
# Random Predictor
def predict_random(context, utterances):
return np.random.choice(len(utterances), 10, replace=False)
# Evaluate Random predictor
y_random = [predict_random(test_df.Context[x], test_df.iloc[x,1:].values) for x in range(len(test_df))]
y_test = np.zeros(len(y_random))
for n in [1, 2, 5, 10]:
print(“Recall @ ({}, 10): {:g}”.format(n, evaluate_recall(y_random, y_test, n)))
Recall @ (1, 10): 0.0937632
Recall @ (2, 10): 0.194503
Recall @ (5, 10): 0.49297
Recall @ (10, 10): 1
Great,
seems to work. Of course we don’t just want a random predictor. Another
baseline that was discussed in the original paper is a tf-idf
predictor. tf-idf
stands for “term frequency — inverse document” frequency and it
measures how important a word in a document is relative to the whole
corpus. Without going into too much detail (you can find many tutorials
about tf-idf on the web), documents that have similar content will have
similar tf-idf vectors. Intuitively, if a context and a response have
similar words they are more likely to be a correct pair. At least more
likely than random. Many libraries out there (such as scikit-learn) come with built-in tf-idf functions, so it’s very easy to use. Let’s build a tf-idf predictor and see how well it performs.
class TFIDFPredictor:
def __init__(self):
self.vectorizer = TfidfVectorizer()
def train(self, data):
self.vectorizer.fit(np.append(data.Context.values,data.Utterance.values))
def predict(self, context, utterances):
# Convert context and utterances into tfidf vector
vector_context = self.vectorizer.transform([context])
vector_doc = self.vectorizer.transform(utterances)
# The dot product measures the similarity of the resulting vectors
result = np.dot(vector_doc, vector_context.T).todense()
result = np.asarray(result).flatten()
# Sort by top results and return the indices in descending order
return np.argsort(result, axis=0)[::-1]
# Evaluate TFIDF predictor
pred = TFIDFPredictor()
pred.train(train_df)
y = [pred.predict(test_df.Context[x], test_df.iloc[x,1:].values) for x in range(len(test_df))]
for n in [1, 2, 5, 10]:
print(“Recall @ ({}, 10): {:g}”.format(n, evaluate_recall(y, y_test, n)))
Recall @ (1, 10): 0.495032
Recall @ (2, 10): 0.596882
Recall @ (5, 10): 0.766121
Recall @ (10, 10): 1
We
can see that the tf-idf model performs significantly better than the
random model. It’s far from perfect though. The assumptions we made
aren’t that great. First of all, a response doesn’t necessarily need to
be similar to the context to be correct. Secondly, tf-idf ignores word
order, which can be an important signal. With a Neural Network model we
can do a bit better.
DUAL ENCODER LSTM
The Deep Learning model we will build in this post is called a Dual Encoder LSTM
network. This type of network is just one of many we could apply to
this problem and it’s not necessarily the best one. You can come up with
all kinds of Deep Learning architectures that haven’t been tried
yet — it’s an active research area. For example, the seq2seq model
often used in Machine Translation would probably do well on this task.
The reason we are going for the Dual Encoder is because it has been reported
to give decent performance on this data set. This means we know what to
expect and can be sure that our implementation is correct. Applying
other models to this problem would be an interesting project.
The Dual Encoder LSTM we’ll build looks like this (paper):
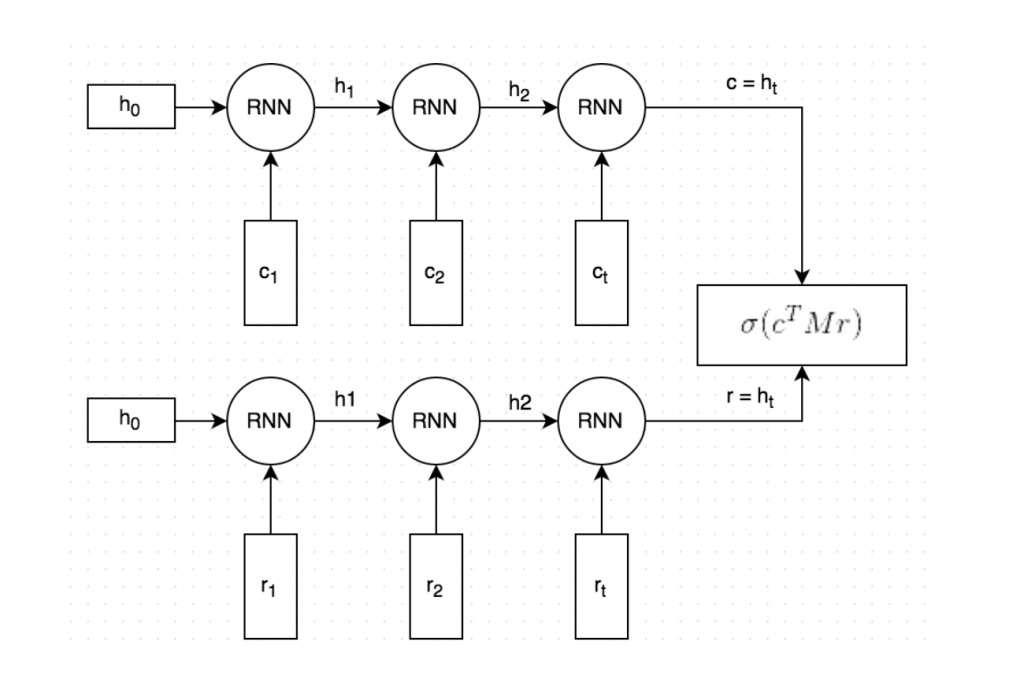
It roughly works as follows:
- Both the context and the response text are split by words, and each word is embedded into a vector. The word embeddings are initialized with Stanford’s GloVe vectors and are fine-tuned during training (Side note: This is optional and not shown in the picture. I found that initializing the word embeddings with GloVe did not make a big difference in terms of model performance).
- Both the embedded context and response are fed into the same Recurrent Neural Network word-by-word. The RNN generates a vector representation that, loosely speaking, captures the “meaning” of the context and response (c and r in the picture). We can choose how large these vectors should be, but let’s say we pick 256 dimensions.
- We multiply c with a matrix M to “predict” a response r’. If c is a 256-dimensional vector, then M is a 256×256 dimensional matrix, and the result is another 256-dimensional vector, which we can interpret as a generated response. The matrix M is learned during training.
- We measure the similarity of the predicted response r’ and the actual response r by taking the dot product of these two vectors. A large dot product means the vectors are similar and that the response should receive a high score. We then apply a sigmoid function to convert that score into a probability. Note that steps 3 and 4 are combined in the figure.
To
train the network, we also need a loss (cost) function. We’ll use the
binary cross-entropy loss common for classification problems. Let’s call
our true label for a context-response pair y. This can be either 1
(actual response) or 0 (incorrect response). Let’s call our predicted
probability from 4. above y’. Then, the cross entropy loss is calculated
as L= −y * ln(y’) − (1 − y) * ln(1−y’). The intuition behind this
formula is simple. If y=1 we are left with L = -ln(y’), which penalizes a
prediction far away from 1, and if y=0 we are left with L= −ln(1−y’),
which penalizes a prediction far away from 0.
For our implementation we’ll use a combination of numpy, pandas, Tensorflow and TF Learn (a combination of high-level convenience functions for Tensorflow).
DATA PREPROCESSING
The dataset
originally comes in CSV format. We could work directly with CSVs, but
it’s better to convert our data into Tensorflow’s proprietary Example
format. (Quick side note: There’s alsotf.SequenceExample but it doesn’t
seem to be supported by tf.learn yet). The main benefit of this format
is that it allows us to load tensors directly from the input files and
let Tensorflow handle all the shuffling, batching and queuing of inputs.
As part of the preprocessing we also create a vocabulary. This means we
map each word to an integer number, e.g. “cat” may become 2631. The
TFRecord files we will generate store these integer numbers instead of
the word strings. We will also save the vocabulary so that we can map
back from integers to words later on.
Each Example contains the following fields:
- context: A sequence of word ids representing the context text, e.g. [231, 2190, 737, 0, 912]
- context_len: The length of the context, e.g. 5 for the above example
- utterance A sequence of word ids representing the utterance (response)
- utterance_len: The length of the utterance
- label: Only in the training data. 0 or 1.
- distractor_[N]: Only in the test/validation data. N ranges from 0 to 8. A sequence of word ids representing the distractor utterance.
- distractor_[N]_len: Only in the test/validation data. N ranges from 0 to 8. The length of the utterance.
The preprocessing is done by the prepare_data.py
Python script, which generates 3 files:train.tfrecords,
validation.tfrecords and test.tfrecords. You can run the script yourself
or download the data files here.
CREATING AN INPUT FUNCTION
In
order to use Tensorflow’s built-in support for training and evaluation
we need to create an input function — a function that returns batches of
our input data. In fact, because our training and test data have
different formats, we need different input functions for them. The input
function should return a batch of features and labels (if available).
Something along the lines of:
def input_fn():
# TODO Load and preprocess data here
return batched_features, labels
Because
we need different input functions during training and evaluation and
because we hate code duplication we create a wrapper called
create_input_fn that creates an input function for the appropriate mode.
It also takes a few other parameters. Here’s the definition we’re
using:
def create_input_fn(mode, input_files, batch_size, num_epochs=None):
def input_fn():
# TODO Load and preprocess data here
return batched_features, labels
return input_fn
The complete code can be found in udc_inputs.py. On a high level, the function does the following:
- Create a feature definition that describes the fields in our Example file
- Read records from the input_files with tf.TFRecordReader
- Parse the records according to the feature definition
- Extract the training labels
- Batch multiple examples and training labels
- Return the batched examples and training labels
DEFINING EVALUATION METRICS
We
already mentioned that we want to use the recall@k metric to evaluate
our model. Luckily, Tensorflow already comes with many standard
evaluation metrics that we can use, including recall@k. To use these
metrics we need to create a dictionary that maps from a metric name to a
function that takes the predictions and label as arguments:
def create_evaluation_metrics():
eval_metrics = {}
for k in [1, 2, 5, 10]:
eval_metrics[“recall_at_%d” % k] = functools.partial(
tf.contrib.metrics.streaming_sparse_recall_at_k,
k=k)
return eval_metrics
Above, we use functools.partial
to convert a function that takes 3 arguments to one that only takes 2
arguments. Don’t let the name streaming_sparse_recall_at_k confuse you.
Streaming just means that the metric is accumulated over multiple
batches, and sparse refers to the format of our labels.
This
brings is to an important point: What exactly is the format of our
predictions during evaluation? During training, we predict the
probability of the example being correct. But during evaluation our goal
is to score the utterance and 9 distractors and pick the best one — we
don’t simply predict correct/incorrect. This means that during
evaluation each example should result in a vector of 10 scores, e.g.
[0.34, 0.11, 0.22, 0.45, 0.01, 0.02, 0.03, 0.08, 0.33, 0.11], where the
scores correspond to the true response and the 9 distractors
respectively. Each utterance is scored independently, so the
probabilities don’t need to add up to 1. Because the true response is
always element 0 in array, the label for each example is 0. The example
above would be counted as classified incorrectly by recall@1because the
third distractor got a probability of 0.45 while the true response only
got 0.34. It would be scored as correct by recall@2 however.
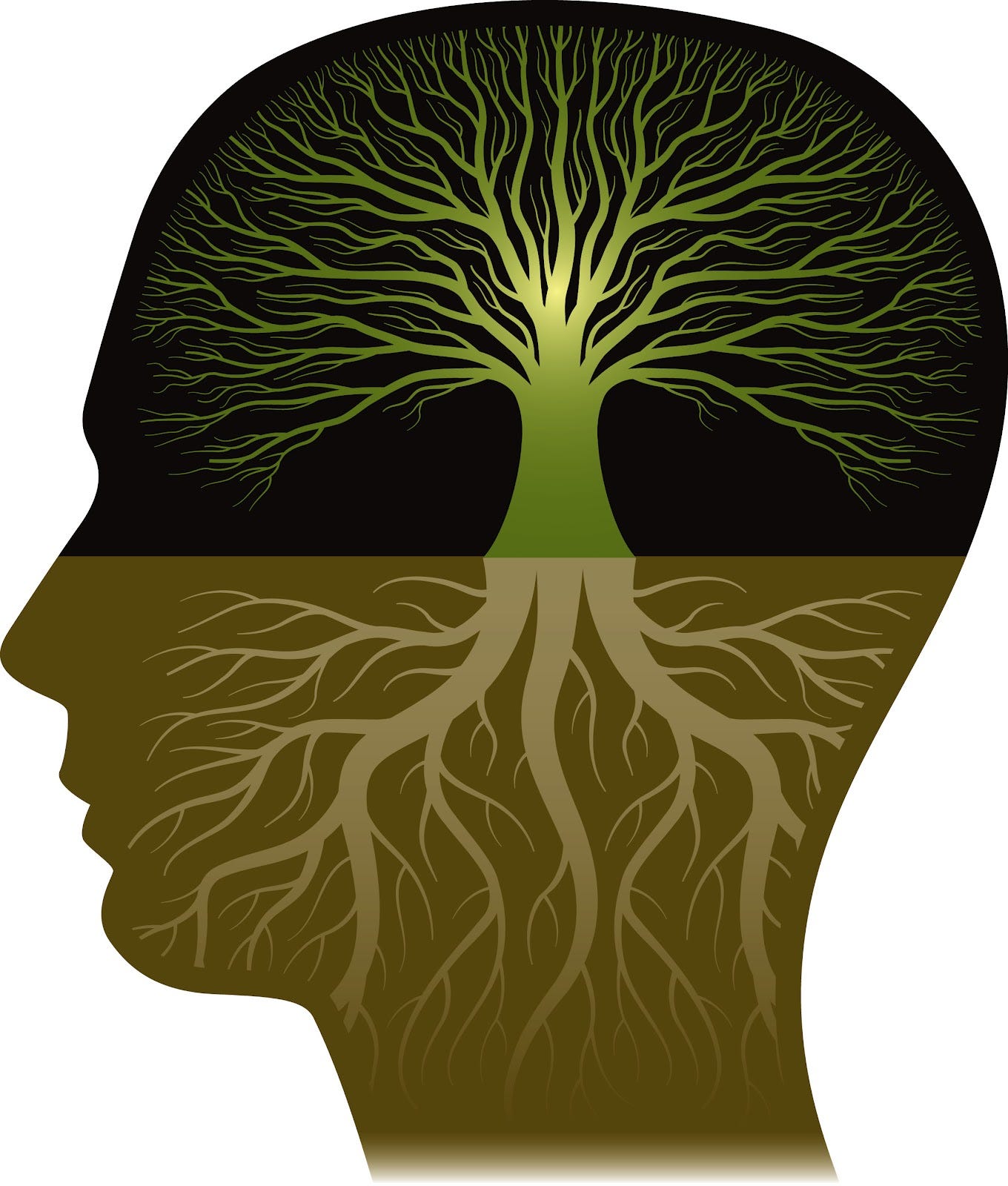
BOILERPLATE TRAINING CODE
Before
writing the actual neural network code I like to write the boilerplate
code for training and evaluating the model. That’s because, as long as
you adhere to the right interfaces, it’s easy to swap out what kind of
network you are using. Let’s assume we have a model functionmodel_fn
that takes as inputs our batched features, labels and mode (train or
evaluation) and returns the predictions. Then we can write
general-purpose code to train our model as follows:
estimator = tf.contrib.learn.Estimator(
model_fn=model_fn,
model_dir=MODEL_DIR,
config=tf.contrib.learn.RunConfig())
input_fn_train = udc_inputs.create_input_fn(
mode=tf.contrib.learn.ModeKeys.TRAIN,
input_files=[TRAIN_FILE],
batch_size=hparams.batch_size)
input_fn_eval = udc_inputs.create_input_fn(
mode=tf.contrib.learn.ModeKeys.EVAL,
input_files=[VALIDATION_FILE],
batch_size=hparams.eval_batch_size,
num_epochs=1)
eval_metrics = udc_metrics.create_evaluation_metrics()
# We need to subclass theis manually for now. The next TF version will
# have support ValidationMonitors with metrics built-in.
# It’s already on the master branch.
class EvaluationMonitor(tf.contrib.learn.monitors.EveryN):
def every_n_step_end(self, step, outputs):
self._estimator.evaluate(
input_fn=input_fn_eval,
metrics=eval_metrics,
steps=None)
eval_monitor = EvaluationMonitor(every_n_steps=FLAGS.eval_every)
estimator.fit(input_fn=input_fn_train, steps=None, monitors=[eval_monitor])
Here
we create an estimator for our model_fn, two input functions for
training and evaluation data, and our evaluation metrics dictionary. We
also define a monitor that evaluates our model every FLAGS.eval_every
steps during training. Finally, we train the model. The training runs
indefinitely, but Tensorflow automatically saves checkpoint files in
MODEL_DIR, so you can stop the training at any time. A more fancy
technique would be to use early stopping, which means you automatically
stop training when a validation set metric stops improving (i.e. you are
starting to overfit). You can see the full code in udc_train.py.
Two
things I want to mention briefly is the usage of FLAGS. This is a way
to give command line parameters to the program (similar to Python’s
argparse). hparams is a custom object we create in hparams.py that holds hyperparameters, nobs we can tweak, of our model. This hparams object is given to the model when we instantiate it.
CREATING THE MODEL
Now
that we have set up the boilerplate code around inputs, parsing,
evaluation and training it’s time to write code for our Dual LSTM neural
network. Because we have different formats of training and evaluation
data I’ve written a create_model_fn
wrapper that takes care of bringing the data into the right format for
us. It takes a model_impl argument, which is a function that actually
makes predictions. In our case it’s the Dual Encoder LSTM we described
above, but we could easily swap it out for some other neural network.
Let’s see what that looks like:
def dual_encoder_model(
hparams,
mode,
context,
context_len,
utterance,
utterance_len,
targets):
# Initialize embedidngs randomly or with pre-trained vectors if available
embeddings_W = get_embeddings(hparams)
# Embed the context and the utterance
context_embedded = tf.nn.embedding_lookup(
embeddings_W, context, name=”embed_context”)
utterance_embedded = tf.nn.embedding_lookup(
embeddings_W, utterance, name=”embed_utterance”)
# Build the RNN
with tf.variable_scope(“rnn”) as vs:
# We use an LSTM Cell
cell = tf.nn.rnn_cell.LSTMCell(
hparams.rnn_dim,
forget_bias=2.0,
use_peepholes=True,
state_is_tuple=True)
# Run the utterance and context through the RNN
rnn_outputs, rnn_states = tf.nn.dynamic_rnn(
cell,
tf.concat(0, [context_embedded, utterance_embedded]),
sequence_length=tf.concat(0, [context_len, utterance_len]),
dtype=tf.float32)
encoding_context, encoding_utterance = tf.split(0, 2, rnn_states.h)
with tf.variable_scope(“prediction”) as vs:
M = tf.get_variable(“M”,
shape=[hparams.rnn_dim, hparams.rnn_dim],
initializer=tf.truncated_normal_initializer())
# “Predict” a response: c * M
generated_response = tf.matmul(encoding_context, M)
generated_response = tf.expand_dims(generated_response, 2)
encoding_utterance = tf.expand_dims(encoding_utterance, 2)
# Dot product between generated response and actual response
# (c * M) * r
logits = tf.batch_matmul(generated_response, encoding_utterance, True)
logits = tf.squeeze(logits, [2])
# Apply sigmoid to convert logits to probabilities
probs = tf.sigmoid(logits)
# Calculate the binary cross-entropy loss
losses = tf.nn.sigmoid_cross_entropy_with_logits(logits, tf.to_float(targets))
# Mean loss across the batch of examples
mean_loss = tf.reduce_mean(losses, name=”mean_loss”)
return probs, mean_loss
The full code is in dual_encoder.py. Given this, we can now instantiate our model function in the main routine in udc_train.py that we defined earlier.
model_fn = udc_model.create_model_fn(
hparams=hparams,
model_impl=dual_encoder_model)
That’s
it! We can now run python udc_train.py and it should start training our
networks, occasionally evaluating recall on our validation data (you
can choose how often you want to evaluate using the — eval_every
switch). To get a complete list of all available command line flags that
we defined using tf.flags and hparams you can run python
udc_train.py — help.
INFO:tensorflow:training step 20200, loss = 0.36895 (0.330 sec/batch).
INFO:tensorflow:Step 20201: mean_loss:0 = 0.385877
INFO:tensorflow:training step 20300, loss = 0.25251 (0.338 sec/batch).
INFO:tensorflow:Step 20301: mean_loss:0 = 0.405653
…
INFO:tensorflow:Results
after 270 steps (0.248 sec/batch): recall_at_1 = 0.507581018519,
recall_at_2 = 0.689699074074, recall_at_5 = 0.913020833333, recall_at_10
= 1.0, loss = 0.5383
…
EVALUATING THE MODEL
After
you’ve trained the model you can evaluate it on the test set using
python udc_test.py — model_dir=$MODEL_DIR_FROM_TRAINING, e.g. python
udc_test.py — model_dir=~/github/chatbot-retrieval/runs/1467389151. This
will run the recall@k evaluation metrics on the test set instead of the
validation set. Note that you must call udc_test.py with the same
parameters you used during training. So, if you trained
with — embedding_size=128 you need to call the test script with the
same.
After training for about 20,000 steps (around an hour on a fast GPU) our model gets the following results on the test set:
recall_at_1 = 0.507581018519
recall_at_2 = 0.689699074074
recall_at_5 = 0.913020833333
While
recall@1 is close to our TFIDF model, recall@2 and recall@5 are
significantly better, suggesting that our neural network assigns higher
scores to the correct answers. The original paper reported 0.55, 0.72
and 0.92 for recall@1, recall@2, and recall@5 respectively, but I
haven’t been able to reproduce scores quite as high. Perhaps additional
data preprocessing or hyperparameter optimization may bump scores up a
bit more.
MAKING PREDICTIONS
You can modify and run udc_predict.py to get probability scores for unseen data. For example python udc_predict.py — model_dir=./runs/1467576365/ outputs:
Context: Example context
Response 1: 0.44806
Response 2: 0.481638
You could imagine feeding in 100 potential responses to a context and then picking the one with the highest score.
CONCLUSION
In
this post we’ve implemented a retrieval-based neural network model that
can assign scores to potential responses given a conversation context.
There is still a lot of room for improvement, however. One can imagine
that other neural networks do better on this task than a dual LSTM
encoder. There is also a lot of room for hyperparameter optimization, or
improvements to the preprocessing step. The Code and data for this tutorial is on Github, so check it out.
Resources:
Denny’s Blogs: http://blog.dennybritz.com/ & http://www.wildml.com/
Mark Clark: https://www.linkedin.com/in/markwclark
Final Word
I
hope you have found this Condensed NLP Guide Helpful. I wanted to
publish a longer version (imagine if this was 5x longer) however I don’t
want to scare the readers away.
As
someone who develops the front end of bots (user experience,
personality, flow, etc) I find it extremely helpful to the understand
the stack, know the technological pros and cons and so to be able to
effectively design around NLP/NLU limitations. Ultimately a lot of the
issues bots face today (eg: context) can be designed around,
effectively.
If you have any suggestions on regarding this article and how it can be improved, feel free to drop me a line.
Let’s Hack Chatbots Together
Creator of 10+ bots, including Smart Notes Bot. Founder of Chatbot’s Life, where we help companies create great chatbots and share our insights along the way.
Want to Talk Bots? Best way to chat directly and see my latest projects is via my Personal Bot: Stefan’s Bot.
Chatbot Projects
Currently,
I’m consulting a number of companies on their chatbot projects. To get
feedback on your Chatbot project or to Start a Chatbot Project, contact me.
No comments:
Post a Comment